While predictive analytics has been a game-changing technology, the rapid advancements in machine learning have taken Artificial Intelligence (AI) analysis to new heights. The traditional approach of predicting future outcomes based on historical data is now being replaced by AI algorithms that can learn and adapt from new data in real-time.
This evolution has not only increased accuracy and efficiency but also opened up endless possibilities for industries ranging from healthcare to finance. With the integration of machine learning into predictive analytics, the future of AI analysis looks even more promising.
The Rise of Predictive Analytics
What is Predictive Analytics?
Predictive analytics is a subset of data analytics that uses statistical techniques, data mining, and machine learning algorithms to analyze historical data and make predictions about future events or outcomes. It combines various fields such as statistics, mathematics, computer science, and business intelligence to forecast trends and patterns using large datasets.
The Advantages of Predictive Analytics
-
Pros:
- Provides insights into customer behavior: Predictive analytics can help businesses understand their customers’ needs and preferences by analyzing their past behaviors.
- Optimizes operational efficiency: By predicting demand for products or services, companies can optimize their inventory management processes.
- Aids decision-making: With accurate predictions based on historical data, organizations can make informed decisions quickly.
- Reduces risk: Predictive analytics can identify potential risks in advance, allowing companies to take necessary measures to mitigate them.
The Limitations of Predictive Analytics
-
Cons:
- Data dependency: The accuracy of predictive models relies heavily on the quality and quantity of available data.
- Not suitable for dynamic environments: Predictive analytics may not be effective in situations where the data is constantly changing and evolving.
- Lack of human interpretation: While predictive models can make accurate predictions, they lack the ability to provide a reasoning behind their decisions.
- Potential bias: If historical data contains biases, it can negatively impact the accuracy of predictive models.
The Emergence of Machine Learning
What is Machine Learning?
Machine learning (ML) is a branch of AI that focuses on developing algorithms and statistical models that enable computer systems to learn from data without being explicitly programmed. It involves training these algorithms with large datasets and continually improving them based on new information.
The Advantages of Machine Learning
-
Pros:
- Improved accuracy: With ML algorithms constantly learning from new data, they can make more accurate predictions than traditional analytics methods.
- Faster decision-making: ML can process and analyze vast amounts of data quickly, allowing organizations to make real-time decisions.
- Uncover complex patterns: Unlike humans, ML algorithms can uncover hidden patterns and relationships in large datasets that would be impossible to identify manually.
- Adaptability: As ML models continue to learn, they can adapt to changes in trends and behaviors, making them suitable for dynamic environments.
The Limitations of Machine Learning
-
Cons:
- Data dependence: As with predictive analytics, ML also relies heavily on vast quantities of high-quality data for training its algorithms.
- Diverse skillset required: Building and maintaining ML models require a diverse skill set, including data science, programming, and domain expertise.
- Black box decision-making: The inner workings of some ML algorithms can be challenging to interpret, making it difficult for humans to understand the reasoning behind their decisions.
The Evolution of AI Analytics
The Role of Big Data in AI Analytics
The rise of big data has played a vital role in the evolution of AI analytics. With the explosion of data from various sources such as social media, sensors, and IoT devices, traditional analytical methods became inadequate. To make sense of this massive amount of data, companies turned to predictive analytics and machine learning techniques.
Predictive analytics helped organizations identify trends and patterns within large datasets, while machine learning enabled them to develop more accurate predictions based on these insights. As technology advancements continue to generate vast amounts of data, AI analytics will only become more critical in extracting valuable insights from this information overload.
The Emergence of Deep Learning
Deep learning is a subset of machine learning that uses artificial neural networks (ANNs) inspired by the human brain’s biological structure. These complex networks are composed of multiple layers capable of processing vast amounts of raw input data and identifying intricate patterns among them.
While deep learning has been around since the 1980s, recent advancements in computing power have led to its widespread use in various industries such as healthcare, finance, and retail. Its ability to process unstructured data like images and text makes it particularly useful for tasks such as image recognition or natural language processing.
The Advantages & Limitations of Deep Learning
-
Pros:
- Highly accurate: Deep learning algorithms can achieve higher levels of accuracy than traditional ML methods.
- Adaptable to new data: As deep learning algorithms continue to learn, they can adapt and improve their performance with new data.
- Handles unstructured data: The ability to process unstructured data gives deep learning an advantage over traditional analytics methods in tasks such as image recognition or natural language processing.
-
Cons:
- Data dependency: Like other ML techniques, deep learning also requires large amounts of high-quality data for training its algorithms.
- Lack of transparency: Interpreting the reasoning behind deep learning decisions can be challenging due to the complex nature of neural networks.
- Computationally expensive: Training deep learning models can be computationally expensive, making it a resource-intensive task for organizations.
The Future of AI Analytics
As we move towards a more technologically advanced world, the future of AI analytics looks promising. With ongoing research and advancements in machine learning and deep learning, we can expect even more accurate predictions and valuable insights from AI analytics.
The integration of AI in various industries is also set to increase significantly. For instance, in healthcare, AI-powered predictive analytics and machine learning are being used to diagnose diseases and predict patient outcomes accurately. In finance, companies are leveraging these technologies to detect fraud and make smarter investment decisions.
There has been a growing focus on explainable artificial intelligence (XAI), which aims to make AI more transparent by explaining how decisions were made. This will address one of the major limitations of black box decision-making in ML algorithms.
New Applications & Use Cases for AI Analytics
As technology continues to advance, we can expect new applications and use cases for AI analytics to emerge. Some potential areas where these technologies could make a significant impact include:
Smart Cities
With the rise of IoT and smart devices, cities are becoming more connected than ever. AI analytics can help city planners analyze data from various sources such as traffic patterns, energy consumption, and weather forecasts to optimize resource utilization and improve overall efficiency.
Risk Management in Insurance
By leveraging predictive analytics and machine learning, insurance companies can better assess risks associated with their policies. This will enable them to offer customized plans and accurately predict potential losses, resulting in more efficient risk management processes.
Cybersecurity
As cyber threats become increasingly sophisticated, traditional security measures may not be enough to protect organizations’ valuable data. AI-powered cybersecurity systems that use predictive analytics and anomaly detection techniques can identify potential attacks before they happen, providing an extra layer of protection against cybercrime.
The Ethical Considerations of AI Analytics
While the advancements in AI analytics have brought numerous benefits, they also raise ethical concerns. As these technologies continue to evolve and integrate into our daily lives, it is crucial to address these issues proactively.
One major concern is biased decision-making by AI algorithms. If trained on biased data sets, ML models can perpetuate existing biases or even create new ones. It is essential for organizations to ensure diversity in their datasets and continually monitor for any signs of bias in their algorithms.
Another issue is privacy violations. Once we delve into the realm of artificial intelligence and its impact on society, it becomes clear that the controversial topic of AI Big Boobs Nude is just one small piece of a much larger puzzle. With the influx of personal data being collected by companies to train their AI models, there is a growing concern about how this information will be used and protected. Regulations like GDPR (General Data Protection Regulation) aim to address these concerns and hold companies accountable for safeguarding user data.
There are concerns about job displacement due to the increased adoption of automation through AI technologies. While some jobs may become obsolete or change significantly, new job opportunities in fields such as data science and AI development are also expected to emerge.
The Impact of AI Analytics on Society
The impact of AI analytics on society has been significant, with its use cases ranging from healthcare and finance to transportation and entertainment. These technologies have the potential to improve efficiency, save lives, and enhance our quality of life significantly.
In healthcare, predictive analytics and machine learning can assist doctors in diagnosing diseases more accurately and identifying treatment options tailored to each patient’s needs. This not only saves time but also improves patient outcomes. To find like-minded goth singles in your area, try using a goth hookup app and connect with potential matches today.
In education, AI-powered adaptive learning platforms can tailor lessons based on individual students’ needs and learning styles. This personalized approach can help students achieve better academic results while reducing teachers’ workload.
Moreover, in transportation, autonomous vehicles powered by AI algorithms could greatly reduce accidents caused by human error. It also has the potential to revolutionize public transport systems by optimizing routes and reducing travel time for commuters.
Recap
From predictive analytics to machine learning and deep learning, the evolution of AI analytics has transformed the way businesses operate. These technologies have enabled organizations to make data-driven decisions faster, optimize operations, and deliver a better customer experience.
As we continue to push the boundaries of technology, there will be even more advancements in AI analytics that will impact various industries positively. However, it is essential to address ethical concerns proactively to ensure these technologies are used responsibly for the greater good of society.
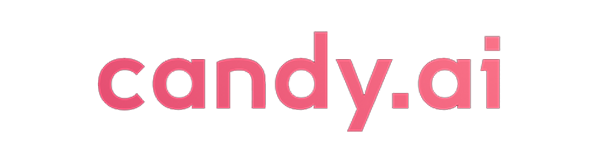
Candy.ai
✔️ Generate AI Porn Images
✔️ Listen To Voice Messages
✔️ Fast Response Time
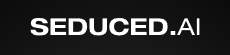
Seduced.ai
✔️ Generate AI Models
✔️ Save & Reuse Girls
✔️ 300 Images Per Month
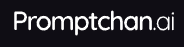
PromptChan.ai
✔️ Completely Free To Test
✔️ Edit Your AI Models
✔️ Make Porn Images (no limit)
What is the purpose of using AI in analytical tasks?
The purpose of using AI in analytical tasks is to improve efficiency, accuracy, and speed. With advanced algorithms and machine learning capabilities, AI can quickly analyze large amounts of data and identify patterns or insights that may be difficult for humans to detect. This allows businesses to make more informed decisions and streamline their operations. AI can continuously learn and adapt, making it a valuable tool for ongoing analysis and optimization.
How does AI technology assist in data analysis?
AI technology has revolutionized the way data is analyzed by using advanced algorithms and machine learning techniques to quickly sift through massive amounts of information. This allows for more accurate and efficient analysis, leading to better insights and decision-making. With AI, businesses and organizations can harness the power of big data like never before.
Can AI accurately interpret and analyze complex data sets?
Yes, AI has the ability to accurately interpret and analyze complex data sets due to its advanced algorithms and machine learning techniques. This allows it to find patterns and insights that may not be immediately apparent to human analysts.
What are some potential benefits of incorporating AI into analytics processes?
Integrating artificial intelligence into analytics processes can bring about numerous advantages, such as enhanced accuracy and speed in data analysis, identification of complex patterns and trends, improved decision-making capabilities, and reduction of human error. AI has the potential to handle large volumes of data at a faster pace, providing valuable insights for businesses to make more informed decisions. Incorporating AI into analytics processes allows for efficiency, innovation, and ultimately better business outcomes.